Creating Efficient Annotated Image Datasets for Business Success
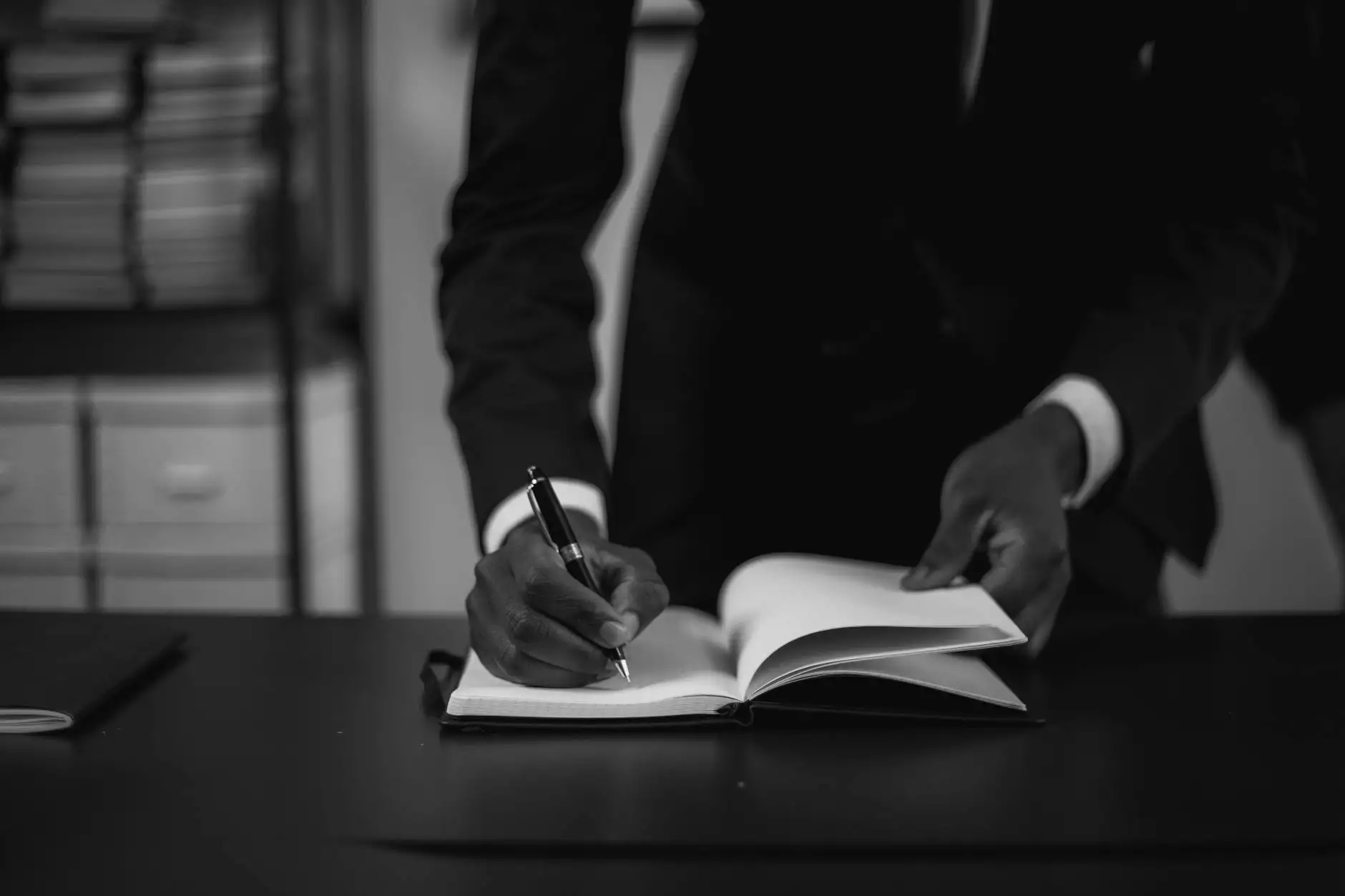
In today's rapidly evolving business landscape, the use of artificial intelligence (AI) and machine learning (ML) technologies has become essential for organizations seeking to maintain a competitive edge. At the heart of these technologies lies one crucial element: annotated image datasets. This article will explore what annotated image datasets are, their significance in various business applications, and how Keylabs.ai can provide cutting-edge tools in Data Annotation and platforms to streamline this process.
Understanding Annotated Image Datasets
An annotated image dataset is a collection of images that have been labeled or tagged to provide context and meaning to each visual element. These datasets serve as the foundation for training machine learning models, enabling systems to recognize and classify objects within images accurately. The quality and accuracy of the annotations significantly impact the performance of AI models, making it imperative for businesses to invest in robust data annotation processes.
The Importance of Annotated Image Datasets in Business
The use of annotated image datasets spans multiple industries, each leveraging this technology to achieve different objectives. Here’s how various sectors utilize these datasets:
- Healthcare: In the medical field, annotated image datasets are crucial for training models that assist in diagnostic imaging, pathology, and radiology. Accurate annotations can help improve the precision of AI systems that detect diseases from X-rays, MRIs, and CT scans.
- Retail: Retail businesses use annotated images for inventory management and customer behavior analysis. By annotating product images, retailers can analyze user interactions, optimize layouts, and enhance customer experiences.
- Automotive: Self-driving car technology relies heavily on annotated datasets for object recognition. These datasets help vehicles identify pedestrians, traffic signs, and obstacles, ensuring safer autonomous navigation.
- Agriculture: Farmers implement image annotation to monitor crop health and yield predictions. Datasets related to plant species and conditions enable the development of predictive models that lead to better agricultural practices.
How Data Annotation Tools Enhance Dataset Quality
To create superior annotated image datasets, businesses must utilize advanced data annotation tools. Here are some critical features these tools offer:
1. High-Quality Annotation Capabilities
Top-tier data annotation tools provide sophisticated features that allow users to annotate images with a high level of precision. This includes bounding boxes, polygonal labeling, semantic segmentation, and image classification, enabling comprehensive understanding and categorization.
2. Collaborative Annotations
Effective annotation processes require collaboration among teams. Using a data annotation platform like Keylabs.ai, multiple users can work on the same dataset simultaneously, ensuring faster turnaround times and consistent quality.
3. AI-Assisted Annotation
AI can expedite the annotation process by providing initial labels or suggestions based on algorithms trained on prior datasets. This feature significantly reduces manual labor while maintaining quality.
Keylabs.ai: Your Partner in Data Annotation
Keylabs.ai offers a comprehensive data annotation platform that simplifies the creation of annotated image datasets. Here’s what sets Keylabs.ai apart:
1. Versatile Annotation Types
With Keylabs.ai, users can choose from numerous annotation types suited for different applications—whether for pixel-wise segmentation, instance segmentation, or bounding box creation, ensuring all needs are met.
2. Scalability and Flexibility
No matter the size of the project, Keylabs.ai can scale to meet your needs. Whether you require a few hundred annotated images or several million, our platform is designed to handle projects of any scope with ease.
3. Advanced Quality Assurance
Quality assurance is vital in data annotation. The platform includes layered feedback systems and validation checks to ensure annotations meet the required standards before they are finalized.
Strategies for Creating Effective Annotated Image Datasets
To ensure your annotated image dataset is effective and suitable for AI applications, consider the following strategies:
1. Define Clear Annotation Guidelines
Establishing clear guidelines for how to annotate images can significantly enhance consistency and accuracy across the dataset. These guidelines should detail every aspect of the annotation process, specific categories, and what constitutes an appropriate label.
2. Leverage Diverse Data Sources
Diversity in your image sources can enhance the robustness of your dataset. Aim to include images that represent various scenarios, lighting conditions, angles, and backgrounds to enable models to generalize better.
3. Regularly Update Datasets
Technology and business environments are continuously evolving, and so should your datasets. Regularly updating the annotated image datasets helps maintain relevance and improve model performance over time.
4. Engage Domain Experts
Involving domain experts in the annotation process can lead to higher accuracy and reliability. These professionals can provide insights that enhance the quality of the annotations, particularly in specialized fields such as healthcare or automotive.
Future Trends in Data Annotation
The field of data annotation is rapidly progressing, driven by advancements in technology and the burgeoning demand for quality datasets. Here are some future trends to keep an eye on:
1. Increased Use of Automation
As AI technologies advance, automation in the annotation process is expected to increase. Automated tools will complement human annotators, making the process faster, more efficient, and less prone to errors.
2. Focus on Explainability
With growing concerns regarding AI transparency, the annotation process will evolve to emphasize explainable AI. This means providing not just labels but also the rationale behind the classifications, building trust in AI systems.
3. Integration with Other Technologies
Data annotation platforms will increasingly integrate with other business systems, such as CRM and ERP solutions, streamlining workflows and enhancing data accessibility across the organization.
Conclusion
In conclusion, annotated image datasets play a pivotal role in the success of AI and machine learning initiatives across industries. By leveraging advanced data annotation tools like those from Keylabs.ai, organizations can enhance the quality of their datasets, streamline processes, and ultimately drive better business outcomes. Embracing these solutions will prepare businesses to thrive in a competitive landscape increasingly defined by data-driven technologies.
Investing in high-quality annotated datasets is no longer an option; it is a necessity for those aiming to exploit the full potential of AI. Partner with Keylabs.ai today to ensure your dataset exceeds expectations and propels your organization towards success.